Automatic assessment of Wikipedia quality in different languages
This article presents an overview of scientific works related to quality assessment of Wikipedia in different languages. Despite the fact that Wikipedia is often criticized for its poor quality, it still is one of the most popular knowledge bases in the world. Currently, this online encyclopedia is on the 5th place in the ranking of most visited sites (after Google, Youtube, Facebook, Baidu). Articles in this encyclopedia are created and edited in about 300 different languages. Currently Wikipedia contains more than 46 million articles about various topics.
Every day the number of articles in Wikipedia is growing. They can be created and edited even by anonymous users. Authors do not need to formally demonstrate their skills, education and experience in certain areas. Wikipedia does not have a central editorial team or a group of reviewers who could comprehensively check all new and existing texts. For these and other reasons, people often criticize the concept of Wikipedia, in particular pointing out the poor quality of information.
Despite this, in Wikipedia you can sometimes find valuable information - depending on the language version and subject. Practically in every language version there is a system of awards for the best articles. However, the number of these articles is relatively small (less than one percent). In some language versions, there are also other quality grades. However, the overwhelming majority of articles have are unevaluated (in some languages more than 99%).
Automatic quality assessment of Wikipedia articles
So, in Wikipedia, many articles do not have quality grades, so each reader should manually analyze their content. The topic of automatic quality assessment of Wikipedia articles in the scientific world is known. Basically, the scientific works describes the most developed language version of Wikipedia - English, which already contains more than 5.5 million articles. In my works I study different language versions of Wikipedia: English, Russian, Polish, Belarusian, Ukrainian, German, French, etc.
Since it foundation and with the growing popularity of Wikipedia, more and more scientific publications on this subject have published. One of the first studies showed that measuring the volume of content can help determine the degree of “maturity” of the Wikipedia article. Works in this direction shows that, in general, higher-quality articles are long, use many references, are edited by hundreds of authors and have thousands of editions.
How do they come to such conclusions? Simply put: comparing good and bad articles.
As already mentioned earlier, in almost every language version of Wikipedia, there is a system of assessing the quality of articles. The best articles are awarded in a special way - they receive a special “badge”. In Russian Wikipedia such articles are called “Featured Articles” (FA). There is another “badge” for articles that slightly below the best ones - “Good articles” (GA). In some language versions, there are other estimates for more “weak” articles. For example, in English Wikipedia there are also: A-class, B-class, C-class, Start, Stub. On the other hand in Russian Wikipedia we can met the following additional grades: Solid, Full, Developed, In development, Stub.
Even on the example of the English and Russian versions, we can conclude that the standards for the grading scale are different and depends on the language. Moreover, not all language versions of Wikipedia have such a developed system of quality assessment of articles. For example, German Wikipedia, which contains more than 2 million articles, uses only two estimates - equivalents for FA and GA. Therefore, often assessments in scientific papers are grouped into two groups: [1][2][3][4][5][6][7]
- ”Complete” - FA and GA grade,
- ”Incomplete” - all other grades.
Data Mining
To build such models, you can use various algorithms, in particular Data Mining. In my works, I often use one of the most common and effective algorithms - Random Forest [1][2][3][4][5][6][7]. There are even studies [4], which compare it with other algorithms (CART, SMO, Multilayer Perceptron, LMT, C4.5, C5.0 and others). Random Forest allows to build models even using variables that correlates with each other. Additionally, this algorithm can show which variables are more important for determining the quality of articles. If we need to get other information about the importance of variables, we can use other algorithms, including logistic regression [13].The results show that there are differences between article quality models in different language versions of Wikipedia[1][2][3][4]. So, if in one language version one of the most important parameters is the number of references (sources), in another language will be more important the number of images and the length of the text.
In this case, the quality is modeled as the probability of referring an article to one of two groups - Complete or Incomplete. The conclusion is made on the basis of analysis of various parameters (metrics): the length of the text, the number of notes, images, sections, links to the article, the number of facts[6], visits, the number of editions and many others. There are also a number of linguistic parameters[5][7], which depend on the considered language. Currently, in total, more than 300 parameters are used in studies, depending on the language version of Wikipedia and the complexity of the quality model. Some parameters, such as references (sources), can be evaluated additionally[14] - we can not only count the quantity, but also assess how well-known and reliable sources are used in the Wikipedia article.
Where to get these parameters?
There are several sources - it can be a backup copy of Wikipedia, API service, special tools and others [12].
To get some parameters, you just need to send a request (query) to the appropriate API, for other parameters (especially linguistic ones) you need to use special libraries and parsers. A considerable part of the time, however, is spent writing your own tools (we’ll talk about this in separate articles).
Are there other ways for quality assessing of Wikipedia articles other than binary?
Yes. Recent studies [8][9] propose the method for estimating articles on a scale from 0 to 100 in a continuous scale. Thus, an article can receive, for example, an estimate of 54.21. This method has been tested in 55 language versions. The results are available on the WikiRank service, which allows you to evaluate and compare the quality and popularity of Wikipedia articles in different languages. The method, of course, is not ideal, but works for locally known topics [9].
Are there ways of assessing the quality of some part of Wikipedia article?
Of course. For example, one of the important elements of the article is the so-called “infobox”. This is a separate frame (table), which is often located at the top right of the article and shows the most important facts about the subject. So, there is no need to look for this information in the text - you can just look at this table. Evaluation of the quality of these infoboxes is devoted to individual studies [2][11]. There are also projects, such as Infoboxes, which allow you to automatically compare the infoboxes in different language versions.
Why do we need all this?
Wikipedia is used often, but the information quality is not always checked. The proposed methods can simplify this task - if the article is bad, then the reader, knowing this, will be more careful in using its materials for decision making. On the other hand, the user can also see in which language the topic of interest is described better. And most importantly, modern techniques allow you to transfer information between different language versions. This means that you can automatically enrich the weak versions of Wikipedia with high-quality data from other language versions[11]. This will also improve the quality of other semantic databases, for which Wikipedia is the main source of information. First of all, this is - DBpedia, Wikidata, YAGO2 and others.
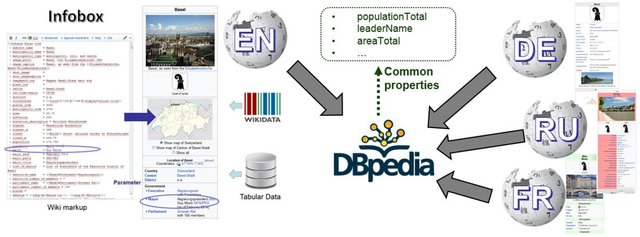
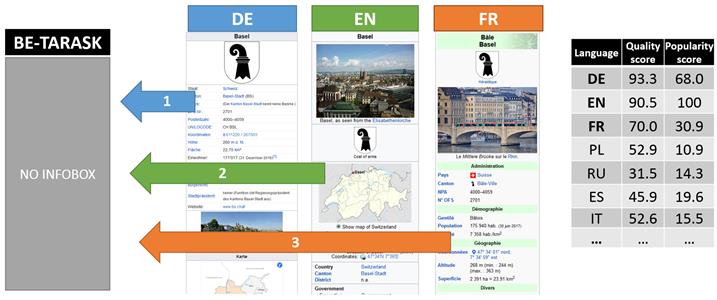
Bibliography
- [1] Lewoniewski, W., Węcel, K., & Abramowicz, W. (2016). Quality and Importance of Wikipedia Articles in Different Languages. In International Conference on Information and Software Technologies (pp. 613-624). Springer International Publishing. DOI: 10.1007/978-3-319-46254-7_50
- [2] Węcel, K., & Lewoniewski, W. (2015). Modelling the quality of attributes in Wikipedia infoboxes. In International Conference on Business Information Systems (pp. 308-320). Springer International Publishing. DOI: 10.1007/978-3-319-26762-3_27
- [3] Lewoniewski, W., Węcel, K., & Abramowicz, W. (2015). Analiza porównawcza modeli jakości informacji w narodowych wersjach Wikipedii. Prace Naukowe/Uniwersytet Ekonomiczny w Katowicach, 133-154.
- [4] Lewoniewski, W., Węcel, K., Abramowicz, W. (2017), Analiza porównawcza modeli klasyfikacyjnych w kontekście oceny jakości artykułów Wikipedii, Matematyka i informatyka na usługach ekonomii, Wydawnictwo UEP Poznań, ISBN 9788374179386
- [5]Khairova, N., Lewoniewski, W., & Węcel, K. (2017). Estimating the quality of articles in Russian Wikipedia using the logical-linguistic model of fact extraction. In International Conference on Business Information Systems (pp. 28-40). Springer, Cham. DOI: 10.1007/978-3-319-59336-4_3
- [6] Lewoniewski, W., Khairova, N., Węcel, K., Stratiienko, N., & Abramowicz, W. (2017). Using Morphological and Semantic Features for the Quality Assessment of Russian Wikipedia. In International Conference on Information and Software Technologies (pp. 550-560). Springer, Cham. DOI: 10.1007/978-3-319-67642-5_46
- [7] Lewoniewski, W., Wecel, K., & Abramowicz, W. (2017). Determining Quality of Articles in Polish Wikipedia Based on Linguistic Features. DOI: 10.20944/preprints201801.0017.v1
- [8] Lewoniewski, W., Węcel, K., & Abramowicz, W. (2017). Relative Quality and Popularity Evaluation of Multilingual Wikipedia Articles. In Informatics (Vol. 4, No. 4, p. 43). Multidisciplinary Digital Publishing Institute. DOI: 10.3390/informatics4040043
- [9] Lewoniewski, W., & Węcel, K. (2017). Relative quality assessment of Wikipedia articles in different languages using synthetic measure. In International Conference on Business Information Systems (pp. 282-292). Springer, Cham. DOI: 10.1007/978-3-319-69023-0_24
- [10] Lewoniewski, W. (2017). Enrichment of Information in Multilingual Wikipedia Based on Quality Analysis. In International Conference on Business Information Systems (pp. 216-227). Springer, Cham. DOI: 10.1007/978-3-319-69023-0_19
- [11] Lewoniewski, W. (2017). Completeness and Reliability of Wikipedia Infoboxes in Various Languages. In International Conference on Business Information Systems (pp. 295-305). Springer, Cham. DOI: 10.1007/978-3-319-69023-0_25
- [12] Lewoniewski, W., Węcel, K., (2017), Cechy artykułów oraz metody ich ekstrakcji na potrzeby oceny jakości informacji w Wikipedii. Studia Oeconomica Posnaniensia 12/2017. DOI: 10.18559/SOEP.2017.12.7
- [13] Lamek, A., Lewoniewski, W. (2017), Zastosowanie regresji logistycznej w ocenie jakości informacji na przykładzie Wikipedii. Studia Oeconomica Posnaniensia 12/2017. DOI: 10.18559/SOEP.2017.12.3
- [14] Lewoniewski, W., Węcel, K., Abramowicz, W., (2017), Analysis of References across Wikipedia Languages. Information and Software Technologies. ICIST 2017. DOI: 10.1007/978-3-319-67642-5_47
Congratulations @lewoniewski! You received a personal award!
Click here to view your Board
Congratulations @lewoniewski! You received a personal award!
You can view your badges on your Steem Board and compare to others on the Steem Ranking
Vote for @Steemitboard as a witness to get one more award and increased upvotes!