Big Data: Unleashing the strength in data
Hello everyone and I hope our day has been stress-free. The concept of big data might not be everyone's favorite but I promise to make it as exciting and educating as possible.
I was using a friend's laptop some months back in the office and we were both Googling on how to resolve a server issue. We were on a site when one adult ads sprung up from the left side of his browser. Well, he looked surprise and clicked the close button of this add and another one came up, lol. Well, I like getting to the root of a problem so when he stepped out, I quickly went to his history and download section of his browser and found out he's been visiting adult sites.
[Image credit: Wikimedia. Creative Commons Attribution-Share Alike 3.0 Unported license.Author: Camelia Boban]
The ads were not as a result of malicious ware or virus attack, Google was just throwing up ads which they think would be "of interest" to him because of his "browsing behavior". Who is Google in this contest? A human? Of course not, it is an automated system. "Something" studied his pattern of browsing and came up with the conclusion that he loves adult content.
What then is big data? There is no one definition that will exhaust the meaning of big data as it is relative. What big data means to google or Microsoft might not be the same with what big data might mean for a small sized or medium sized organization. But one thing is very common in the meaning of big data; it is a collection of huge and tremendous amount of data. It is a term used to describe the techniques and technologies applied in the collection, processing and making reasonable decisions based on huge collection of data.
History of Big Data
Some of us are just waking up to discover they're not as "private" as they think, we're in digital age so what do you expect? The idea of big data has been a very old one . The concept of analyzing collections of data in search of an answer or a solution has been around for a very long time.
The first recorded problem which arose as a result of overwhelming data was the issue which faced America's Census Bureau in 1880. The department collected data so huge that the stipulated time to process it was 8 years! This was data for just one year census and the estimated that it would even take longer than 10 years to process the data for the following year. A machine which made use of punch cards was later invented by an employee of the same department, Herman Hollerith, which reduced the data processing job estimated for ten years to just three years.
Similar problem was encountered during the world war two and this time the problem was how to decipher Nazi codes. To do this, a machine was invented which was able to analyze the patterns of messages that were intercepted from German opposition. This machine which was termed Colossus could process five characters per second and this highly aided the ICT team tremendously. A very important figure in development of such machine was the Von Neumann who was also the first man to design the architecture of modern computer.
{Today's internet emerged from DoD's ARPA (Advanced Research Project Agency). Image credit: Wikimedia. Creative commons license. Author: Defence Systems Agency]
The development of modern internet was also a major building block for big data. Modern internet emerged immediately the legacy ARPANET (Advanced Research Project Agency Network) was shut down due to its inability to keep up with modern computer communication requirements such as speed and resilience. ARPANET (a division of Department of Defense, DoD) was said to have started late 1969 and the first computer communication was between host computer in the University of California, Los Angeles and another host computer in Stanford University.
How does big data differ from traditional data handling?
Big data handles data processing differently and it would be impractical to compare the amount of data generated in the internet today to that generated 2 years ago. One of the major source of these huge data is the interactive web, the Web 2.0 which started since 2003. The web 2.0 made collaboration and interaction so easy and in real-time.
The three Vs of big data simply described how big data differs from traditional data systems and ultimately described its characteristics and these vectors are;
- Volume
- Velocity and
- Variety
Volume
[The three Vs of big data. Credit: Wikimedia. Creative Commons Attribution-Share Alike 4.0 International license. Author: MuhammadAbuHijleh]
The volume is one of the first characteristics of big data and I'm really trying hard to explain this vector using simple terms. Now let's take Facebook for example, China is one of the most populated country I know of, but do you know that Facebook has far more user accounts than China's population?
This morning alone, I have updated my FB status with three photos and I'm sure millions or billions of other users has done so in the past few hours and statistics have it that Facebook has over 250 billion images and over 2.5 trillion posts as of 2016!
The Facebook data is a tip of the iceberg from the stables of web 2.0, let’s consider the internet of things which is increasingly gaining popularity. A humidity sensor connected to the internet with the function of sending the level of moisture in a farmer’s farm remotely to him and is designed to send such data every minute could generate over half a million data points per year. What about thousands of sensors connected to so many machines in just one factory?
Let’s also consider the amount of data generated by our smartphones. Take for instance an android To-do app, Wonderlist, as of the time of writing this post, the app currently has over 10 million downloads from the Google’s Playstore and could have up to 9 million active users. These active users certainly have more than one active lists stored in free storage in the “cloud”, hence, there’s no gainsaying the amount of data these users must’ve garnered since the app was made available in the store. Yet, this is just an app, there are millions of other apps, from IOS environment to Windows to Linux, to mention but a few.
The Web 2.0 technologies include blogs, flickr, real simple syndication (RSS), Google docs, Twitter, wikis, etc. The amount of video uploaded on YouTube in the past 6 months can be comparable to the amount of video uploaded in both 2011 and 2012. We are also yet to consider the amount of data collection available in many establishments and industry like the information and communication sector, health sector, energy sector and so on. This is the volume aspect of the big data.
Velocity
Velocity in this contest means how fast data enters the big data system. In the explanation of the volume vector, I pointed out about three data source which are Facebook, IoT and our smartphones. The three images I uploaded for my status was followed up with some posts. If Facebook has billions of users I bet they would receive millions or billions of images per day and also millions or billions of posts in a day. The post I made since I joined Facebook in 2008 are still accessible today and I guess I will still be able to access future multimedia I and my friends uploaded. Which means that the statistics provided above about Facebook having trillions of posts as of 2016 could have possibly doubled if not tripled.
I use the Facebook scenario extensively because it is something all of us can relate with. These huge data received by Facebook will still be processed, stored and definitely, with the ability of retrieval.
One of the methods applied in combating cyber-attacks in today’s networks involves studying streams of data as it passes firewalls. Though encryption is steadily making this approach impractical, malicious packets can be detected by studying patterns of packet flow. The velocity vector describes how data moves within a big data system.
Variety
Our traditional database system usually consists of rows and columns. But how do you classify data that are varied very widely? Take for instance, the database of student information for a university, this could simply be setup and could consist of student names, department, registration number, etc. These data can easily be grouped and the information they are conveying are very obvious.
How about getting a performance rating of U. S President, Donald Trump from comments sent in from millions of people around the world? How do you group such data? Many establishments makes use of twitter feed in finding out how satisfied their customers are with their goods or services. The above examples are from human perspective, what about the data in our smartphones and computers? Many apps connected to the big data system formats their data differently making data from such source impossible to be fitted into a traditional database system. The variety vector of big data describes how unstructured and varied data being handled by big data are.
[The above information is a valid dataset for a big data system. Credit: Wikimedia. Creative Commons Attribution 3.0 Unported license. Author: Photo.iep]
Big Data Analytics
With a concise understanding of the three Vs I explained above, one can easily see that big data has to deal with tremendous amount of data and these data are increasing by the day and most times, processing of such data is done in real-time. Even though data is increasingly becoming complex, one of the enabling features of data which is storage is also incredibly becoming cheaper. And as such, companies and industries can easily store huge data about such as information from sensors, business interactions, customers reactions, etc and present these data to big data for processing.
The process of converting these high volumes of varieties (unstructured) of data into useful information or into data structures that can easily be analyzed by analysts (human or machine) is known as big data analytics. The idea of data analytics has been around for ages but big data brings new possibilities to the table and the most obvious one is speed.
[Clustered computing showing distributed storage and high availability (redundant links). Credit: Wikimedia. Creative Commons Attribution-Share Alike 2.5 Generic license. Author: Georgewilliamherbert]
Before data analytics can be carried out within the big data system, the data must’ve been ingested into the system using some specialized solutions like the Apache Chukwa which can sum up data from user application servers, server logs, etc., and a process known as extract, transform and load is executed which performs initial data sorting and micro analysis.
When data has been ingested into the system, such data needs to be stored. Storage of such data might sound very simple but one term that is always associated with big data is clustered computing since it is almost impossible to handle such data using one system.
With clustered computing, issues could arise such as resource allocation, redundancy for high availability, and how scalable the whole system could be. This shows that the traditional storage system will not work for big data. A widely used approach for handling ingested data in the big data system is through the distributed file system. This allows huge amount of data to be written across many systems within a cluster. Once storage has been achieved, data analytics and presentation can then be carried out.
One of the widely used method of processing and analyzing data in the big data system is the Map Reduction method. Here, the collected data is split into smaller units with each unit assigned to a node within a cluster, after each unit has been processed, the resulting data is reshuffled producing even better results which can be used to carry out calculations to produce a generalized result. This process is known as batch processing.
[Batch processing can easily help you narrow down a search based on many variables. Credit: Wikimedia. Creative Commons Attribution-Share Alike 4.0 International license. Author: Remper]
Batch processing is used if the ingested data requires large amount of calculations and computation but many a times, ingested data is required to be processed immediately and the result used to carry out a function also immediately. This is known as Real-time processing. A very popular data analytics used for real-time processing is the Stream processing and as the name implies, it processes data as it enters the system in streams.
Summary
One of the things I used to tell myself when trying to learn about everything I could in the IT world is that "ignorance is no excuse". When you're busy deciding whether to buy into the idea of big data, other companies are already using it to push sales, predict product performance and easily digest customer complaints.
One of the move some sports betting sites use in predicting many matches with high degree of accuracy is studying patterns and winning history of clubs. I had a very useful battery saver app in my phone sometimes back and one impressive property of that app is that it studies my pattern of phone usage for a period of 7 days after which it can accurately control my phone's processes and incredibly prolong my phone's battery.
Big data deals with tremendous amount of data. It analyzes such data to discover patterns, correlations and so much information within a very short period of time and in most times these processing are done real-time.
REFERENCES
- Volume, velocity, and variety: Understanding the three V's of big data -zdnet
- An Introduction to Big Data Concepts and Terminology -digitalocean
- Big data -Wikipedia
- Big data analytics -sas
If you write STEM (Science, Technology, Engineering, and Mathematics) related posts, consider joining #steemSTEM on steemit chat or discord here. If you are from Nigeria, you may want to include the #stemng tag in your post. You can visit this blog by @stemng for more details. You can also check this blog post by @steemstem here and this guidelines here for help on how to be a member of @steemstem. Please also check this blog post from @steemstem on proper use of images devoid of copyright issues here.
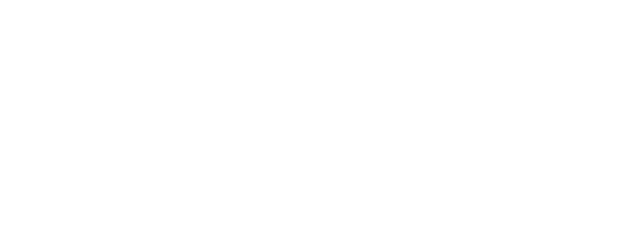
Helo @henrychidiebere.
Big data is such a huge concept. Initially, I really wondered what it was going by the title of this post but thank goodness, you made everything so easy to understand. And your summary of the concept is rich and telling.
Do have a nice weekend.
Regards.
@eurogee of @euronation and @steemstem communities
If there's something I want desperately for my readers, it's getting first hand information about the trends in IT industry. It's not easy breaking it down though but it gives me joy knowing that people get to understand the concept even in my struggle to simplify things.
Yeah big data is gaining grounds and it doesn't get simpler than this. Thanks @eurogee
Good to know ✌️
Big data
Machine learning
There a lot to learn
'The Big Data'
Fascinating concept I'd say. Thanks for the insight. Nice article up here! Kudos