Machine Learning, a journey to doomsday and the rise of terminators or not?
So much buzz about Artificial Intelligence, but what about the rock upon which Artificial Intelligence is laid? Let's steem!
Learning, similar to intelligence, cuts across a vast range of processes that is hard to define accurately. The dictionary definition of learning include phrases such as "gain knowledge", "skill by studying", " experience something". Machine Learning isn't a new field. Many thanks to the new advances in computer technologies, it is workable to apply algorithms to loads of data that grow and change at tremendous speed. These complex systems are able to adjust themselves as well as make it possible to perform various tasks like predict a time for increase demand/supply in a business or drive vehicles autonomously.
Image credit: Wikimedia Commons, SPL_Team_B-Human,_RoboCup_2016 BY Peter Schulz CC 4.0
What is Machine Learning?
Incontestably, this field of #STEM is fast gaining momentum, but what is it basically? What is machine learning?Machine Learning is the science concerned with getting machines to learn and make decisions for themselves. It enables computer to act without being explicitly programmed.
We know that zoologists and psychologists study learning in animals and humans respectively, but here we are concerned with learning in machines. There are many similarities between animal and machine learning , fairly most of the techniques employed in machine learning resulted from the efforts of psychologist to make accurate their theories of human and animal learning through computational models, likewise also the study of machine learning will as well shine light into other areas of biological learning.
In what ways have machines learnt to learn?
Computer Science far back as the 90s had laid major theoretical backgrounds for machine learning mainly through the development of neutral networks. This is more like designing circuits to simulate the way neurons are connected to the brain in humans. The way in which the brain with its billions neutrons and each neutron connected to up to 1000 more is the prevalent metaphor for the organization of machine learning programs. Instead of the conventional old approach that looks for the best way possible to solve a problem like for example ( to determine the best sequence of moves to checkmate during a chess game), machine learning is concerned with letting the system reason out the rules from scratch.Machine learning is a broad field, making it continually divided into different specialities, among which we can name these two:
• Supervised machine learning: It finds usage in most applications. It requires governing or human intervention for the creation of tags in historical data so that machines can predict a likely result from them. An example is in prediciton of possible time of increase demand/supply in a business/economic system.
• Unsupervised machine learning: finds less usage compared to the former. It uses historical data that has not been tagged. The motive is to find patterns from the data analysis itself. A common use is in the differentiation of customers with similar tastes for a promotional campaign.
In reality, what has machine learning achieved so far?
With the approach of deep learning, an approach in which stacks of neurons are hierarchically arranged to recognise objects, machines can now defeat human champions of games that require intuition, computation or both. Remember that Google's "AlphaGo AI" and "AlphaZero AI" defeated world best "Go" player and Chess player respectively a couple of months ago.Image credit: wikimedia Commons, Chess_gameboard BY Levente Fulop CC 2.0
Also the current most advanced humanoid robot named Sophia who trolled Elon Musk during a live interview at a conference has been given citizenship in Saudi Arabia.
Though not yet perfect, Sophia can sufficiently reply to questions from humans and ask questions likewise.
Image credit: Wikimedia Commons, Sophia_(robot) BY ITU Pictures CC BY 2.0
Other areas transformed by machine learning include;
• Virtual personal assistant ( in homes for smart speakers such as Google home & Amazon echo, for smartphones such as Apple Siri and Samsung bixby).
• Face recognition
• Malware/virus filtering
• Video surveillance
• Transport system & Traffic prediction
• Customer needs prediction/ product recommendation
• Search engines results
• Self driving cars.
Limitations
1• Time lag in learning: It is not possible to make immediate and precise predictions with a machine learning system since it relies or learn via historical data.The bigger the data and the longer a machine is exposed to these data, the more reliable/better it will perform.
2• Diagnosis of error and correction: Machine learning systems are prone to errors. Once they make errors, diagnosing and correcting them can be difficult because it requires going through the fundamental complexities of the algorithms and its underlying associated processes.
3• Verification problems: Machines cannot think or reason like humans naturally since they rely on statistical truths rather than literal truths. In circumstances that are not included in the historical data, it is challenging to prove with utmost certainty that a machine learning system prediction is suitable for all scenarios and conditions.
4• Prediction Limitations: Machine algorithms do not understand "context" as regarding to matters. They are susceptible to biases and hidden tricks depending on the data used in training them.
If machine learning together with AI, 3D printing, and nanotechnology are going to be doing all the work, what the heck will humans do 9 - 5 ??
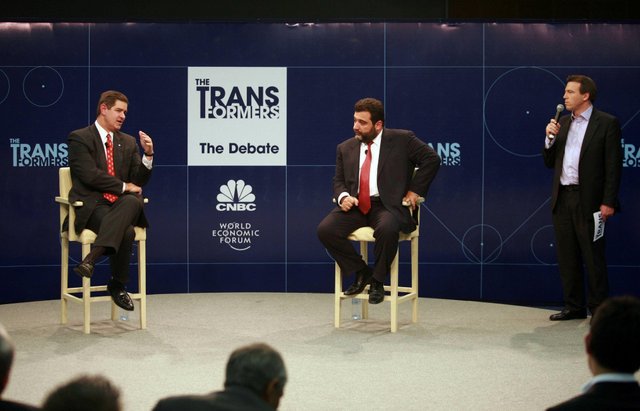
This was the limelight, of a panel at the World Economic Forum held 23-26 January, 2018 in Davos titled "putting jobs out of work". Read full report at Huffpost. This and many more are some of the questions machine learning and other technological advancements throw at us.
Can machine learning ever surpass human learning? For me, at the moment, it's a NO since a machine figures out statistical truth other than literal truth by relying on historical data it is fed with. What are your thoughts?
References/Further reading
TechemergenceQNils, J.N. "Introduction to Machine Learning." Standford University
Would you mind following me back? https://steemit.com/@a-0-0
Educative post but some of your images seem not to be from free sources. You might want to fix that in your future posts. Feel free to join the steemstem discord channel and learn more about how to write more acceptable stem posts.
Thanks. Fixed!
Your Post Has Been Featured on @Resteemable!
Feature any Steemit post using resteemit.com!
How It Works:
1. Take Any Steemit URL
2. Erase
https://
3. Type
re
Get Featured Instantly & Featured Posts are voted every 2.4hrs
Join the Curation Team Here | Vote Resteemable for Witness