Micro-content and the future of Chatbots
Chatbot also known as talkbot, chatterbot or Artificial Conversational Entity, is a computer program which conducts a conversation via auditory or textual methods. Such programs are often designed to convincingly simulate how a human would behave as a conversational partner, thereby passing the Turing test.
Artificial Conversational Entity are typically used in dialog systems for various practical purposes including customer service or information acquisition. Some chatterbots use sophisticated natural language processing systems, but many simpler systems scan for keywords within the input, then pull a reply with the most matching keywords, or the most similar wording pattern, from a database.
In 1950, Alan Turing’s famous article “Computing Machinery and Intelligence” was published, which proposed what is now called the Turing test as a criterion of intelligence. This criterion depends on the ability of a computer program to impersonate a human in a real-time written conversation with a human judge, sufficiently well that the judge is unable to distinguish reliably on the basis of the conversational content alone between the program and a real human.
This technology got traction in the 1960’s; the aim was to see if chatbot systems could fool users that they were real humans. However,chatbot systems are not only built to mimic human conversation, and entertain users. In this paper, I will investigate other applications where chatbots could be useful such as education, information retrival, business,and e-commerce. A range of chatbots with useful applications, including several based on the ALICE/AIML architecture.
Just as people use language for human communication, people want to use their language to communicate with computers. I would agree that the best way to facilitate Human Computer Interaction (HCI) is by allowing users “to express their interest, wishes, or queries directly and naturally, by speaking, typing, and pointing”.
This was the driver behind the development of chatbots. A chatbot system is a software program that interacts with users using natural language. The purpose of a chatbot system is to simulate a human conversation; the chatbot architecture integrates a language model and computational algorithms to emulate informal chat communication between a human user and a computer using natural language thereby allowing the computer to interact freely with it’s human user on the set boundaries of topic, service or unique environment.
Initially, developers built and used chatbots for fun, and used simple keyword matching techniques to find a match of a user input, such as ELIZA.
The notoriety of Turing’s proposed test stimulated great interest in Joseph Weizenbaum’s program ELIZA, published in 1966, which seemed to be able to fool users into believing that they were conversing with a real human.
The seventies and eighties, before the arrival of graphical user interfaces, saw rapid growth in text and natural-language interface research. Since that time, a range of new chatbot architectures have been developed, such as: MegaHAL, CONVERSE, ELIZABETH , HEXBOT and ALICE.
With the improvement of data-mining and machine-learning techniques, better decision making capabilities, availability of corpora, robust linguistic annotations/processing tools standards like XML and its applications, chatbots have become more practical, with many commercial applications .
ALICE
A.L.I.C.E. (Artificial Intelligence Foundation) is the Artificial Linguistic Internet Computer Entity, which was first implemented by Wallace in 1995. Alice’s knowledge about English conversation patterns is stored in AIML files. AIML, or Artificial Intelligence Mark-up Language, is a derivative of Extensible Mark-up Language (XML). It was developed by Wallace and the Alicebot free software community from 1995 onwards to enable people to input dialogue pattern knowledge into chatbots based on the A.L.I.C.E. open-source software technology.
AIML consists of data objects called AIML objects, which are made up of units called topics and categories. The topic is an optional top-level element, has a name attribute and a set of categories related to that topic. Categories are the basic unit of knowledge in AIML. Each category is a rule for matching an input and converting to an output, and consists of a pattern, which matches against the user input, and a template.
With the first chatbot system having been invented in the 60s and now serving as the basis for modern bots. What’s different is that they’re being cast into new platforms like Facebook Messenger, Slack, Outlook, and Skype, and augmented more and more with artificial intelligence and machine learning. Chatbots – or chatterbots – used to be exclusively embedded into smart devices, but can now be expanded across mediums, whether chat or voice based. The emphasis on business use cases that dominated the spotlight in the Spring of 2016 has awakened more and more businesses to the idea of chatbot integration into their daily business processes.
Certainly they will revolutionize search, and reduce not only wait times to reach a human but the cost of the workforce needed to serve the customer, with a side of novelty. They can be designed for anything from automated customer support to e-commerce, interactive experiences, and entertainment. They are continuously being re-vamped to streamline the customer experience and meet the hastening human need for speed.
ChatBots can, among many other tasks:
• check shipping orders
• order food, a car, or flowers
• check weather and traffic
• give you the news
• access product info
• help you shop
• manage your calendar
• play your favorite music
• give answers to questions depending on the span of their vocabulary
• book travel and hotels…
This is clearly a wave that will not subside in the near future, but what is the best way forward? What do chatbots need to be successful?
For me I believe the answer is Microcontent. Usability adviser Jakob Nielsen originally referred to microcontent as small groups of words that can be skimmed by a person to get a clear idea of the content of a Web page. He included article headlines, page titles, subject lines and e-mail headings. Such phrases also may be taken out of context and displayed on a directory, search result page, bookmark list, etc.
To deliver that content though, chatbots need to be able to access the right content at the right time, quickly and accurately. They need to keep step with the micro-moments that make up our lives: breaking content into small bites – microcontent and making it semantically rich.
With a microcontent, all of the information customers seek that can be found in help content, product documentation, and user manuals is structured hierarchically, which is the way the mind works. This creates an easily navigated decision tree that can lead to the best problem-solving information and heightened customer success.
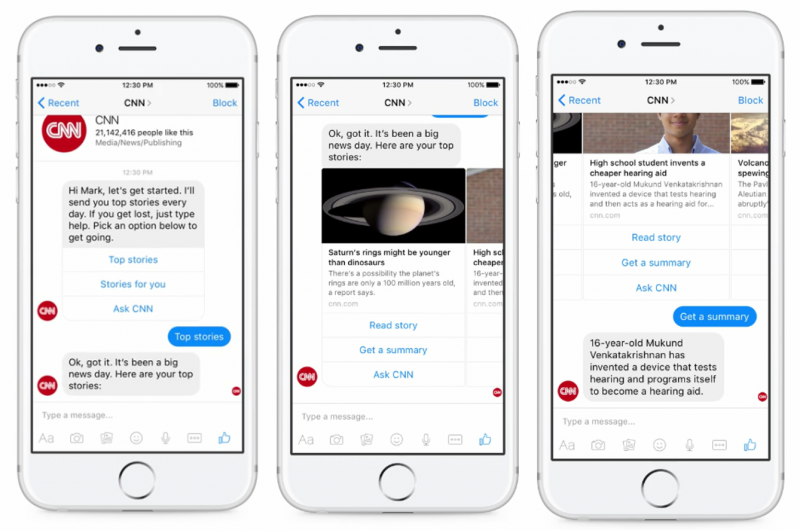
Search engines favor semantically rich micro-content, making it highly valuable for SEO and especially ideal for mobile sites where parsing a full-length PDF user manual is simply out of the question. Micro-content enables bots that can cross platforms (like Alexa) to easily and instantly pull content directly from a company’s website for the user, lowering effort, because it’s the most accurate semantically rich content. Breaking out dense help content into bite-sized pieces that are structured to be searchable and machine readable is another way to make bots better. Further, user activity and inquiries can be tracked, providing rich user data to the company and informing machine learning as the bot matures. This forms one of the key pillars in the future of Chatbot and if a well developed Micro-content algorithm is implemented to a chatbot the we can truly experience real computer to human interaction.

www.siftweir.com